Unveiling the Reliability of GPT-4: A Deep Dive into AI's Conversational Powerhouse
The Limitations of GPT-4
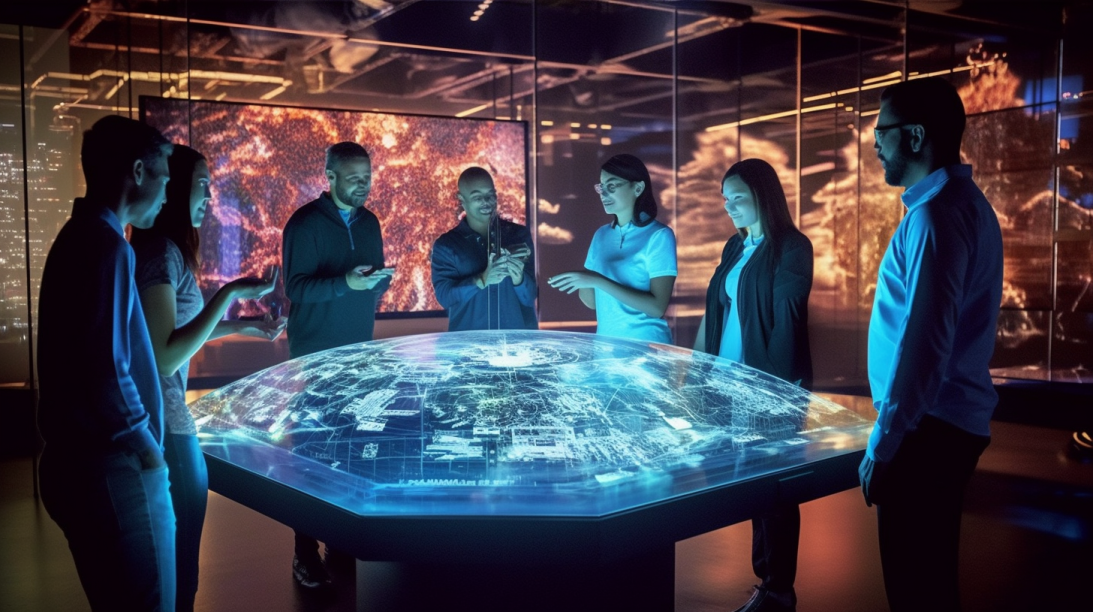
In the realm of artificial intelligence, OpenAI's GPT-4 has sparked a revolution, captivating tech enthusiasts, researchers, and industry professionals with its impressive abilities and broad application possibilities. Amidst the excitement, a critical aspect that often gets overshadowed is GPT-4's reliability. In this article, we delve into the fascinating world of GPT-4, exploring its reliability, understanding its workings, and learning how to harness its power for dependable conversations.
Understanding GPT-4 and Its Functioning
GPT-4, short for Generative Pre-trained Transformer 4, is a language model developed by OpenAI. This intelligent model uses machine learning to generate human-like text based on the input it's given. Trained on a diverse range of internet text, GPT-4 doesn't have specifics about which documents were part of its training set and cannot access any proprietary databases or personal data unless explicitly inputted during a conversation. It's important to note that the model doesn't have the ability to remember or learn from user interactions. Its responses are generated based on the input it receives and what it learned during its training.
Unraveling the Reliability of GPT-4
Reliability, in this context, refers to a model's capacity to produce consistent, accurate, and unbiased responses. It's not just about producing grammatically correct sentences or seemingly intelligent responses; it's about ensuring that the generated content is factually correct, fair, and reliable across various contexts and topics.
Recent research from Microsoft has shed light on the reliability of large language models like GPT-4. This study decomposed reliability into four facets: generalizability, fairness, calibration, and factuality. They found that by using appropriate prompts, GPT-4 can significantly outperform smaller-scale supervised models in these four aspects.
Generalizability
Generalizability refers to a model's ability to apply learned knowledge to new, unseen domains. By crafting carefully structured prompts, GPT-4 can generalize out-of-domain, meaning it can apply its training on vast text datasets to generate responses in areas it has not explicitly been trained on.
Fairness
Fairness, in AI terms, refers to the model's ability to produce unbiased outputs. The research showed that by balancing the demographic distribution in the prompts, social biases in the responses could be significantly reduced.
Calibration
Calibration pertains to the correlation between a model's predicted probabilities and the observed probabilities. By fine-tuning the prompts, one can calibrate GPT-4's language model probabilities, making it a reliable prediction tool.
Factuality
Factuality involves ensuring that the model's outputs are accurate and true to reality. To this end, the study demonstrated that GPT-4's knowledge could be updated effectively using specific prompts, thereby improving the factuality of its responses.
The Power of Prompts
A 'prompt' in the context of GPT-4 refers to the input given to the model, which it uses to generate a response. GPT-4 is a 'few-shot learner', which means it can understand the task it needs to perform based on a few examples included in the prompt. Understanding how to effectively use prompts can greatly improve the reliability of GPT-4 in practical applications. By crafting prompts that guide the model towards desired outputs, users can ensure that only reliable sources are used, or ask for the sources from which the information is derived.
The Limitations of GPT-4
While this research highlights GPT-4's potential in these four aspects, it's also important to note the limitations of this AI model. As pointed out in an opinionpiece by the Communications of the ACM, GPT-4 has several limitations - reliability, interpretability, accessibility, speed, and more. While these limitations may be addressed in future iterations of GPT, none are trivial and some are very challenging to fix.
Despite these challenges, the key to effectively using GPT-4 lies in understanding these limitations and developing strategies to mitigate them. One such strategy involves the use of prompts.
Harnessing the Power of Prompts
A 'prompt' in the context of GPT-4 refers to the input given to the model, which it uses to generate a response. GPT-4 is a 'few-shot learner', which means it can understand the task it needs to perform based on a few examples included in the prompt.
Understanding how to effectively use prompts can greatly improve the reliability of GPT-4 in practical applications. By crafting prompts that guide the model towards desired outputs, users can ensure that only reliable sources are used, or ask for the sources from which the information is derived.
Here are a few example prompts that illustrate this:
- Asking GPT-4 to generate information based on reliable sources:Prompt: "As an AI language model, please provide a summary of the climate change impacts on global health, based on reliable scientific sources."
- Asking GPT-4 to cite its sources:Prompt: "What is the current state of research on Alzheimer's disease treatments? Please cite the sources for the information provided."
- Guiding GPT-4 to avoid certain types of sources:Prompt: "Provide a summary of the latest advancements in quantum computing. Please avoid using blogs or non-academic sources in your response."
Remember, GPT-4 doesn't have direct access to databases or the internet. When it generates information, it's based on the patterns and data it learned during training. The model doesn't "know" the source of its information in the way humans understand knowledge. However, the prompts above guide the model to generate responses that are likely to align with reliable, verifiable information.
In Conclusion
OpenAI's GPT-4 is a powerful tool that brings a new level of sophistication to natural language understanding and generation. However, its reliability is a complex issue, dependent on various factors such as the model's generalizability, fairness, calibration, and factuality.
While research has shown that GPT-4 can be highly reliable when properly prompted, it's also crucial to be aware of the model's limitations. As users and developers of this technology, we should strive to understand and mitigate these limitations to make the most out of GPT-4's capabilities.
In the end, the power of GPT-4 lies not only in its intricate algorithms and extensive training data but also in the hands of its users. By understanding how to craft effective prompts, we can guide GPT-3 to produce reliable, accurate, and fair responses, paving the way for a new era of reliable AI-powered conversations.